Rule of Sixteen
The “Rule of Sixteen” is a simple approximation used by option traders to quickly get an idea of the potential move a particular underlying security might make. As a quick example, suppose it’s a day before expiration and you’re trying to determine how concerned you should be about covering a position of out of the money options. The Rule of Sixteen says that with an implied volatility of 32 there is about a 2/3rds chance that the underlying will move 2% or less in that one remaining day. One out of every three days the underlying will move more than 2%.
The approximation is easily calculated by dividing the implied volatility by 16. The result is simply the “de-annualized” volatility, or in other words, the standard deviation of the daily normalized price returns. Using this result (the standard deviation) and basic statistics, a trader should then know that there is a 68.27% (approx. 2/3rds) chance that the underlying will move less than one standard deviation, or 2% in this example. There is also a 95.45% chance that the underlying will move less than 4% in this example, or two standard deviations.
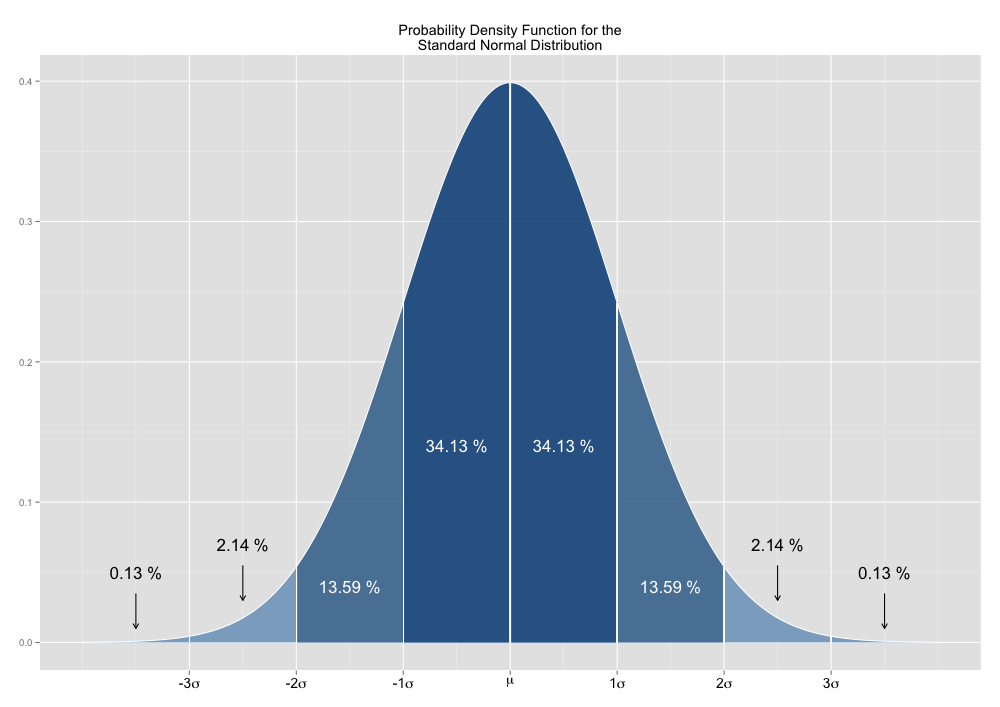
Interpreting The Rule of Sixteen is just a simple matter of analyzing the standard deviation in the context of the standard normal distribution. However, the reason that this approximation is called The Rule of 16 rather than The Rule of Twelve, or The Rule of Nineteen has to do with the process of “annualizing” the standard deviation.
When calculating the historical volatility of an underlying security the standard deviation of the normalized price returns is multiplied by a ratio to calculate the annualized volatility. This “annualizing” ratio is calculated as the square root of the number of trading days in a year divided by the number of days in the price return. So for a normal year, where there are 252 trading days and the historical price returns have been calculated daily, this annualizing ratio would be the square root of 252 divided by 1, or 15.87. This ratio is sufficiently close to 16 and is the reason that it’s called The Rule of Sixteen.
While The Rule of Sixteen can be a handy tool for quickly approximating the potential range of movement in an underlying security, there is a much larger issue that traders should be aware of beyond just the mathematical simplifications that go into this tool. The interpretation of The Rule of Sixteen is typically based upon the analysis of standard deviation in the context of the normal distribution. This is an assumption that can lead to potentially disastrous results.
When analyzing the distribution of price returns for a particular security it’s not uncommon to discover that the higher moments diverge significantly from that of the standard normal distribution. Often the price returns will exhibit skewness and/or kurtosis well in excess of the standard normal distribution. While skewness can be problematic, significant amounts of excess kurtosis can be absolutely devastating from a risk management standpoint. Ignoring excess kurtosis, and the fat-tails associated with it, can lead to prices range estimates that are significantly understated.
So while The Rule of Sixteen can be a quick and helpful way to estimate a probable range of prices, it’s significant limitations should be well understood before use.